Concepts on Machine Learning
Reference book: Hands-On Machine Learning with Scikit-Learn, Keras & TensorFlow by Aurelien Geron - 2nd Edition.
Machine Learning is the “field of study that gives computers the ability to learn without being explicitly programmed.” - Arthur Samuel, 1959.
Machine learning is great for:
Problems for which existing solutions require a lot of fine-tuning or a long list of rules.
Complex problems for which using a traditional approach yields no good solution.
Fluctuating environments where an ML system can adapt to new data.
Help to gather insights about complex problems and large amounts of data.
Types of ML Systems
Trained with human supervision or not. Types of human supervised learning are: Supervised, unsupervised, semi-supervised and reinforcement learning.
Whether or not they can learn incrementally on the fly - online versus batch learning.
Whether they work by simply comparing new data points (instance-based) or instead by detecting patterns in the training data. With these patterns, they are then able to build a predictive model (model-based).
A combination of the above methods.
Supervised Learning Algorithms
k-Nearest Neighbours
Linear Regression
Logistic Regression
Support Vector Machines (SVMs)
Decision Trees and Random Forests
Neural Networks.
Some neural network architectures can be unsupervised, such as auto-encoders and restricted Boltzmann machines. They may also be semi-supervised, such as in deep belief networks and unsupervised pre-training.
Linear Regression
In linear regression problems, we usually use a cost function that measures the distance between the linear model’s predictions and the training example. The objective here is to minimise this distance between the predictions and the training values.
With linear regression, it finds the values for y = mx + c.
Batch Learning
In batch learning, the system is incapable of learning incrementally. It must be trained every time using all available data. This is called offline learning.
Online Learning
With online learning, the system is trained incrementally by feeding it data instances sequentially; either individually or in small groups called mini-batches. Each learning step is quick, so the system can learn about the new data on the fly as it arrives.
It is good for data that is received continuously and it needs to adapt rapidly and autonomously.
It is also a good option when computing resources are expensive.
An important parameter for online learning is the learning rate - how quickly it adapts to changing data.
The disadvantage of online learning is that if bad data is fed to the system, the system’s performance will gradually decline.
The Maths behind Machine Learning
There is a lot of maths involved in machine learning, if you want to some understanding of how software is employed in machine learning.
The maths involved are briefly described in this section.
Linear Algebra
线性代数 xian4 xing4 dai4 shu4
The study of vectors and matrices. ML requires quantifying the similarity between two vectors. Its sub topics are:
Systems of Linear Equations
Matrices
Solving Systems of Linear Equations
Vector Spaces
Linear Independence
Basis and Rank
Linear mappings
Affine Spaces
Analytic Geometry
解析几何 jie3 xi1 ji1 he2
The understanding of similarity and distances.
Matrix Decomposition
Some operations on matrices such as matrix decomposition are extremely useful in machine learning.
Probability Theory
可能性 ke3 neng2 xing4
Being able to identify signal from noise is important in ML. Probability theory helps in this.
Vector Calculus
向量 xiang4 liang4 微积分 wei1 ji1 fen1
Many optimisation techniques require the concept of understanding gradient, a measure of the direction in which to search for a solution.
Optimisation
使完善 shi1 wan2 shan4
Involves finding the maxima and minima of functions.
Forward Propagation with Perceptrons
The diagrams below the concept behind perceptrons work in machine learning.
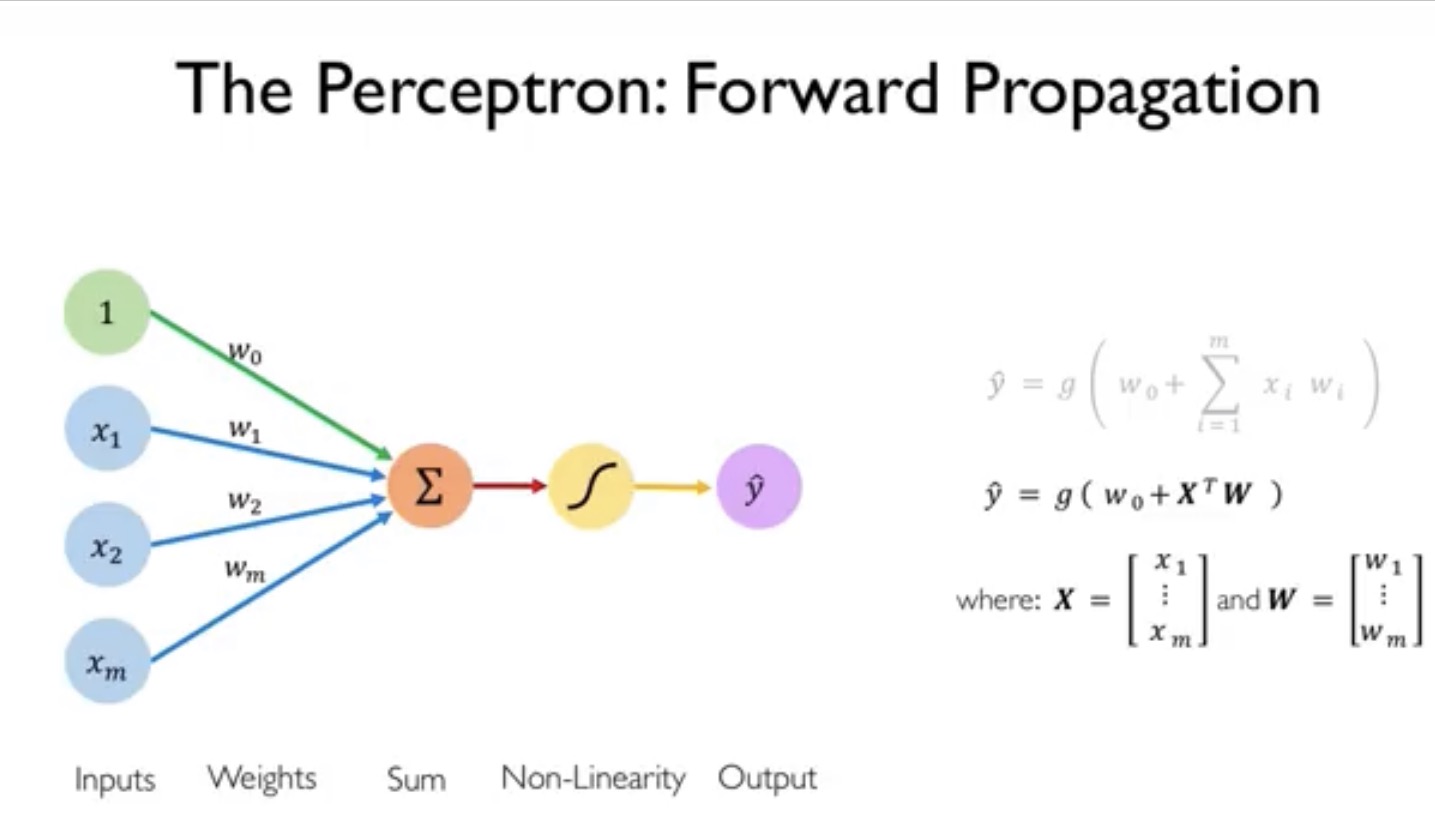
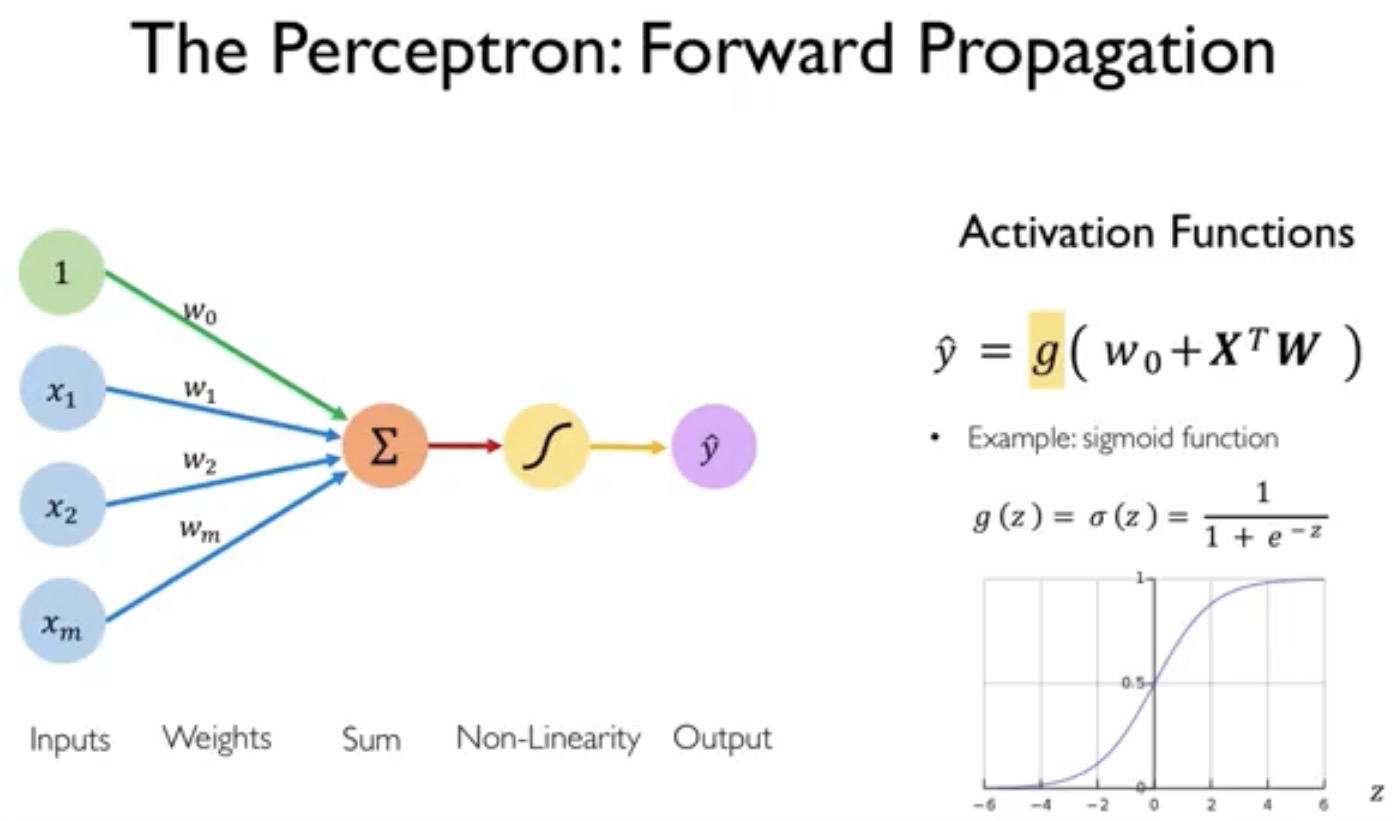
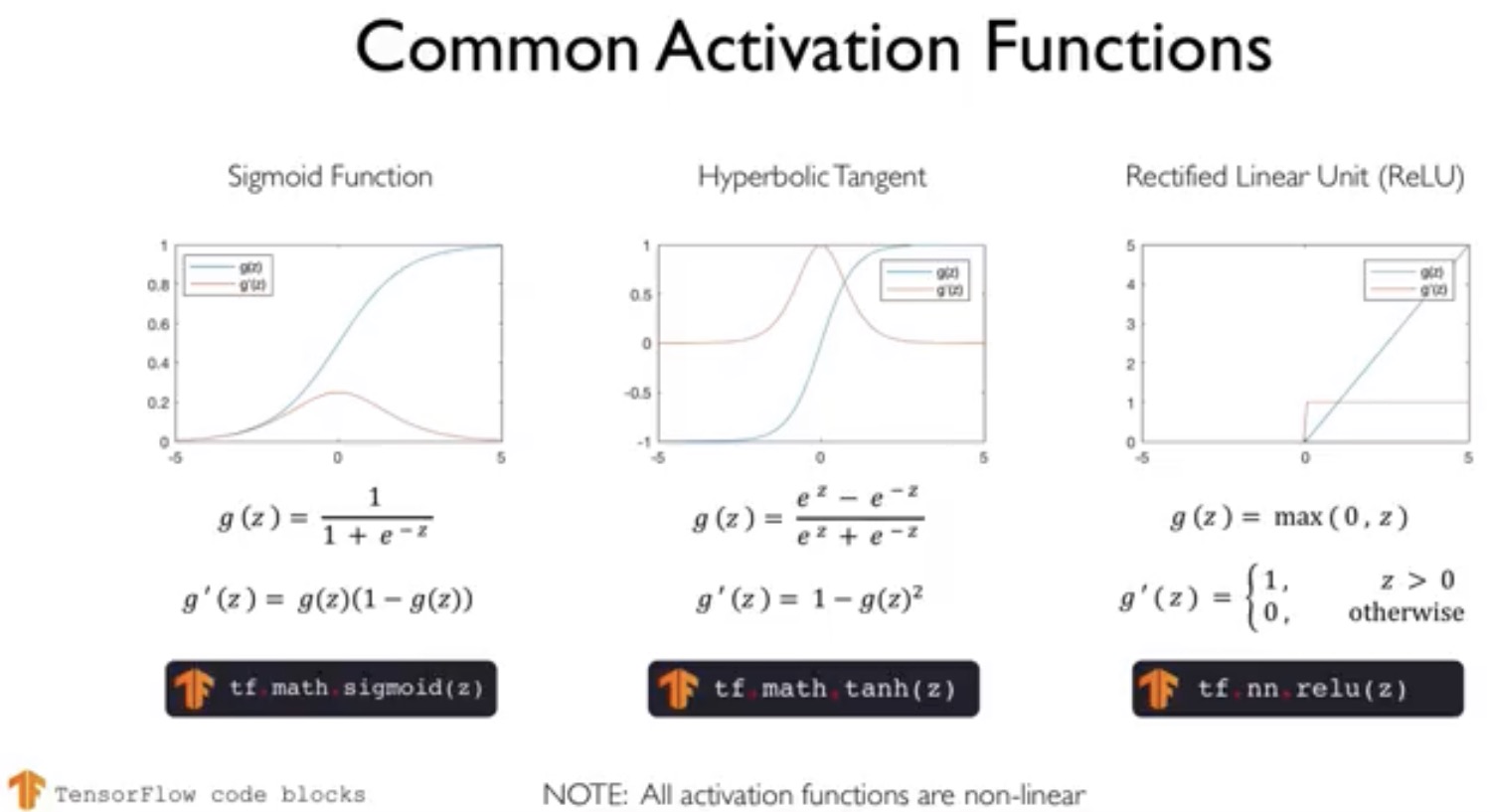